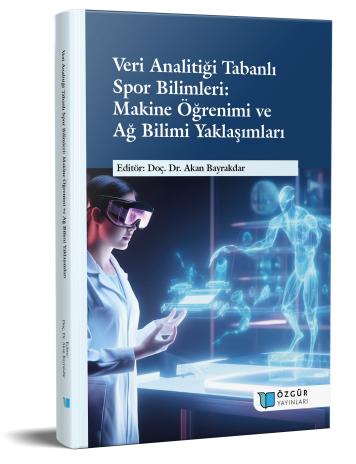
Network Science in Data-Driven Football Analysis
Chapter from the book:
Bayrakdar,
A.
(ed.)
2025.
Data Analytics Based Sports Science: Machine Learning and Network Science Approaches.
Synopsis
This study provides a comprehensive overview of the use of network science methods in soccer analysis. In recent years, with the rise of data-based analysis in sports science, network science has emerged as an important tool for understanding complex interactions in team sports. By modeling the passing and position changes between players through nodes and links, network science allows the strategic structure of a team to be evaluated. Especially in dynamic sports such as football, players' passing networks and centrality measures play a critical role in understanding the team's offensive and defensive organizations.
Passing networks in soccer allow for the visualization of interactions between players. In these networks, nodes represent players and links represent passes. Analyzing passing patterns provides important information for determining the team's playing strategies and player roles. Centrality measures (degree, betweenness, closeness, eigenvector) allow to quantitatively assess players' roles within the team and their contribution to the game. Through these analyses, team strengths and weaknesses can be identified, player performances can be objectively evaluated and strategic decision-making processes can be supported.
Network science techniques are also used to analyze player position and understand formation dynamics. Temporal variations of players' positions on the field provide insight into how the team's playing structure is shaped. Studies show that data obtained using network science can provide valuable information for training planning and opponent analysis. In this context, more widespread and effective use of network science in football analytics will contribute to a more accurate analysis of team performances.