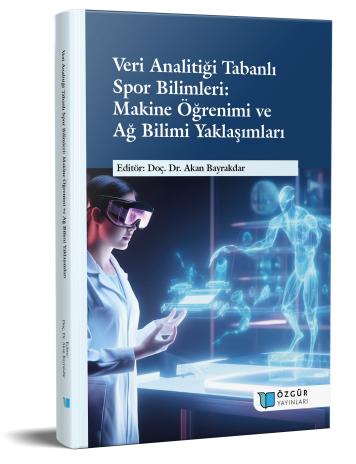
Evaluation of Dance Movements with Machine Learning
Chapter from the book:
Bayrakdar,
A.
(ed.)
2025.
Data Analytics Based Sports Science: Machine Learning and Network Science Approaches.
Synopsis
Work at the intersection of dance and machine learning is enabling the innovative merging of art and technology. In this review, we investigate how artificial intelligence (AI) and machine learning (ML) techniques are used in dance movement recognition, classification and choreography production. In particular, it is observed that deep learning-based models provide high accuracy rates in dance movement quality assessment and style recognition processes.
The use of models such as ML-2DMC-FCM-GWO and Graph Convolutional Adversarial Networks (GCAN) in dance movement recognition and analysis processes contributes to making performance analysis more reliable. In addition, AI-supported systems make dance education more accessible and effective. For example, the DanceGen and PirouNet models offer innovative approaches to choreography production processes, making artistic creation processes more efficient.
The combination of virtual reality (VR) and multimodal learning techniques adds a new dimension to the analysis of dance performances. Thanks to these technologies, dance movements can be analyzed multidimensionally and performance evaluations become more objective. In the future, it is proposed to develop AI-based models to better understand and classify the emotional dimension of dance. This study aims to present the current applications and future research directions of dance and AI integration.